For part 3 of our AI in Fintech section of our AI Notes series, we zoom into more industries where we see the greatest value being unlocked: fraud detection and analytics. We take a global overview and breakdown, before going into the applications of these technologies, both through partnerships and in-house proprietary tools, in consumer and business lending. Revisit Part 2 on Personal Finance
Fraud Detection and Analytics Market Overview
The booming global market for fraud detection and prevention is valued at US$63B in 2023 and is projected to surge to US$178B by 2030. This rapid growth is fueled by an increase in online transactions, which in turn has resulted in a rising tide of cybercrime. With e-commerce retailers facing a potential loss of over US$20B per year due to online fraudulent activities, the demand for robust anti-fraud systems has never been greater.
AI for Fraud Detection and Anti-Money Laundering
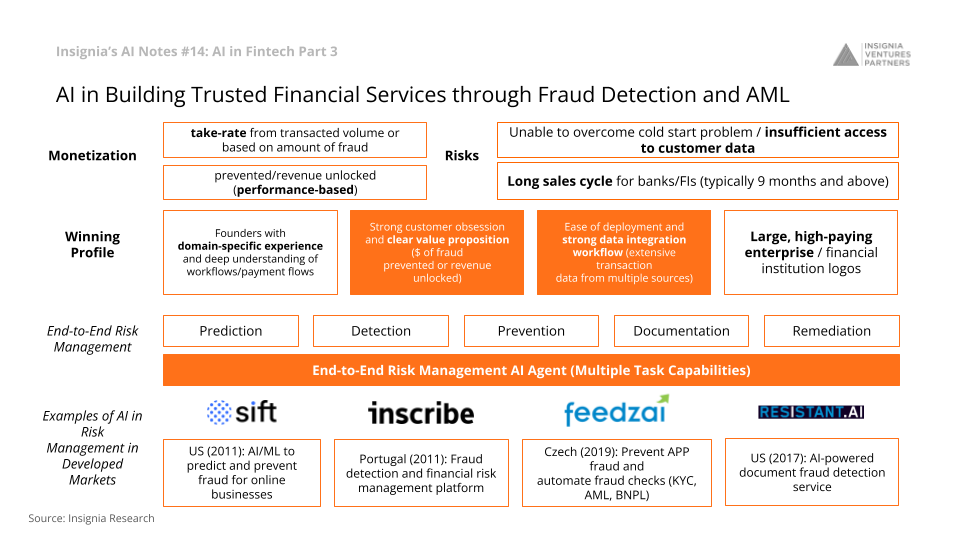
AI in Building Trusted Financial Services through Fraud Detection and AML
Business Model
Companies usually operate on a performance-based business model, either taking a percentage from the transacted volume or based on the amount of fraud prevented or revenue unlocked.
Winning Profile
To be successful in this field, founders should have domain-specific experience and understand the intricacies of payment flows. Companies also need to have a strong data integration workflow and should be able to quickly onboard large, high-paying enterprises and financial institutions.
Risks
Despite the promise, companies in this sector face challenges like the ‘cold start’ problem, where there is insufficient access to customer data, and the long sales cycles typical of banks and financial institutions.
Notable Companies
- Sift, US (2011): Utilizes AI/ML to predict and prevent fraud for online businesses.
- InscribeAI, US (2017): Offers an AI-powered document fraud detection service.
- Feedzai, Portugal (2011): Has been successful due to strong data integration workflows and a continual investment in R&D. The company serves large banks, fintechs, e-commerce merchants, and payment processors, highlighting the scalability and versatility of their solutions.
Applications in Southeast Asia
Verihubs, founded in Indonesia in 2019, specializes in AI-based verification system services, ranging from social media screening to biometric verification technology. Vivi Mandasari, COO of Verihubs, emphasizes that their primary clientele are financial institutions and fintech companies seeking to build a secure digital financial ecosystem.
“Currently our technology is mostly needed by financial institutions or FinTech…as one of the main “backbones” in order to build a safe and confidential digital financial ecosystem for their stakeholders…We have some kind of use case for the hospitality and education [establishments]. So all our products are more adapted for other industries right now, but for the main use cases, it’s still for KYC…”
Implications of AI for Fraud Detection and AML in Lending across the Entire Data Workflow for Risk Management
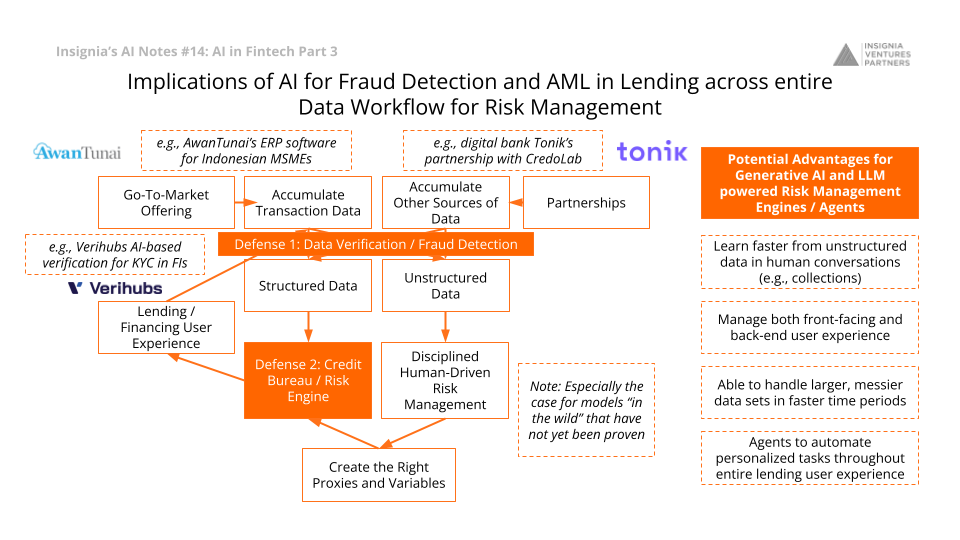
Implications of AI for Fraud Detection and AML in Lending across entire Data Workflow for Risk Management
For Consumers and Banks
According to our podcast with Greg Krasnov, CEO of digital bank Tonik, there’s enormous value to be extracted from the data lakes generated by digital activity. They have partnered with Credolab, which specialize in creating scorecards from device data, offer predictive behavioral insights that far exceed human intuition.
“Now I think it’s more advantageous in a way that we’re swimming in this massive sea of data. So, how to make good use of the data and really create proper systems for the data lake itself, the data warehouses, the machine learning and AI stuff that sits on top of that, that cranks it for looking for predictiveness. So all of that is a very exciting experience for me. Because you can really squeeze a lot of value out of this data. For example, we’re using device data quite actively. And I was a co-founder of another company before Tonik, called Credolab, which actually specializes in this space.
All they do all day long is take the stuff that’s in your smartphone, pull it out, and create a scorecard from it. It’s actually an incredibly data-rich environment that is behaviorally extremely predictive if you know how to crunch it. So Credolab is one of our partners at Tonik as well, and we’re using their data. We’re using telecom data. We’re using the data of customer behavior in our app: which channels he has come in through, how did he work with the app, what’s he doing with his account, etc.”
We actually decided that this is such a strategic area for us, especially with the advance of AI, that we’re looking for a senior person to bring into the team as a head of AI and machine learning.
For Businesses
Dino Setiawan, CEO of MSME supply chain financing platform AwanTunai, argues on our podcast that AI models require nuanced human training, especially in the absence of massive, clean data sets. A blend of structured and unstructured data is critical in developing models that can surpass human performance in risk management.
“This is why we believe that humans need to train AI engines. For instance, when a borrower is verified before loan disbursement, the credit officer will validate their name, address, and borrowed amount. These are three variables in a structured data model, and it’s almost like a binary input. However, the credit officer’s call with the borrower generates unstructured data that is crucial to risk management.
An experienced underwriter listening to the call can determine whether the borrower’s responses are unusual or suspicious. If the underwriter detects anything suspicious, they ask more questions. This unstructured data doesn’t end up in a 50,000-variable model, and that’s where the human element comes in. We always validate the risk process or outcome, whether it’s possible with humans.”
This is why understanding risk management is critical before building science models that can surpass human performance. And to get the right set of variables and proxies, experienced humans need to guide AI engine development.
Potential Advantages for Generative AI and LLM Powered Risk Management Engines / Agents
- Faster learning from unstructured data in human conversations, like debt collection calls.
- Ability to manage both front-facing and back-end user experiences efficiently.
- Capacity to process larger, messier data sets in shorter periods, offering a more comprehensive view of risk.
In summary, AI’s role in fraud detection and anti-money laundering is not merely disruptive; it’s foundational for building a trust ecosystem in financial services. As more businesses and consumers transact online, the AI models that keep these activities secure will become an integral part of the global financial infrastructure.
Follow our LinkedIn page for the latest updates on our weekly AI insights and other insights in Southeast Asia’s innovation landscape.
Author’s Note: This article was written with insights and research from our investment team. Reach out to them if you’re building in this space out of Southeast Asia and would like a deeper conversation.
Stay tuned for the final part of AI in fintech next week!
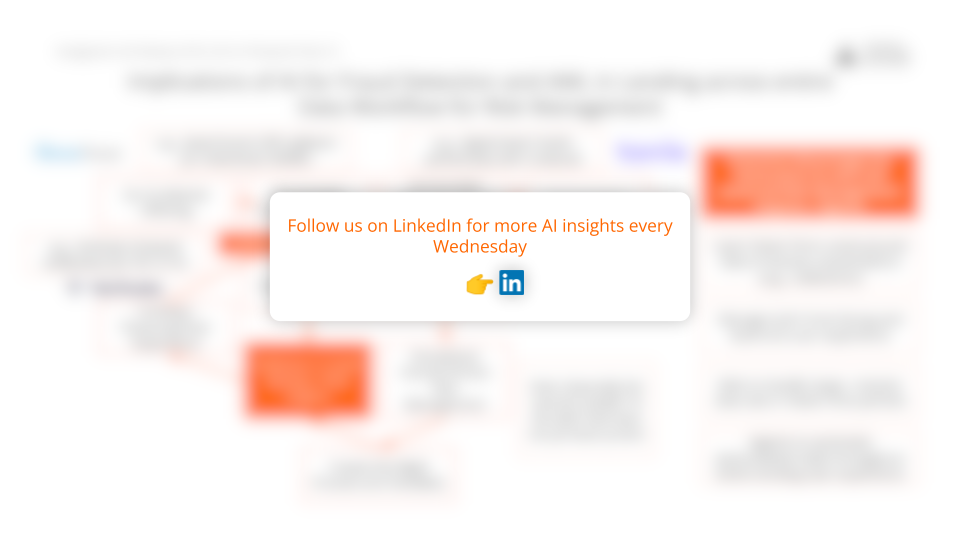
Follow us on LinkedIn for more AI insights every Wednesday
Paulo Joquiño is a writer and content producer for tech companies, and co-author of the book Navigating ASEANnovation. He is currently Editor of Insignia Business Review, the official publication of Insignia Ventures Partners, and senior content strategist for the venture capital firm, where he started right after graduation. As a university student, he took up multiple work opportunities in content and marketing for startups in Asia. These included interning as an associate at G3 Partners, a Seoul-based marketing agency for tech startups, running tech community engagements at coworking space and business community, ASPACE Philippines, and interning at workspace marketplace FlySpaces. He graduated with a BS Management Engineering at Ateneo de Manila University in 2019.