While Gen AI is accelerating faster than any prior technological development (autonomous vehicles took 15 years to become highly autonomous while Gen AI took 5 years to reach a similar stage), whether or not they can be maintained and adopted at scale is another question altogether.
See the previous 15 AI Notes here
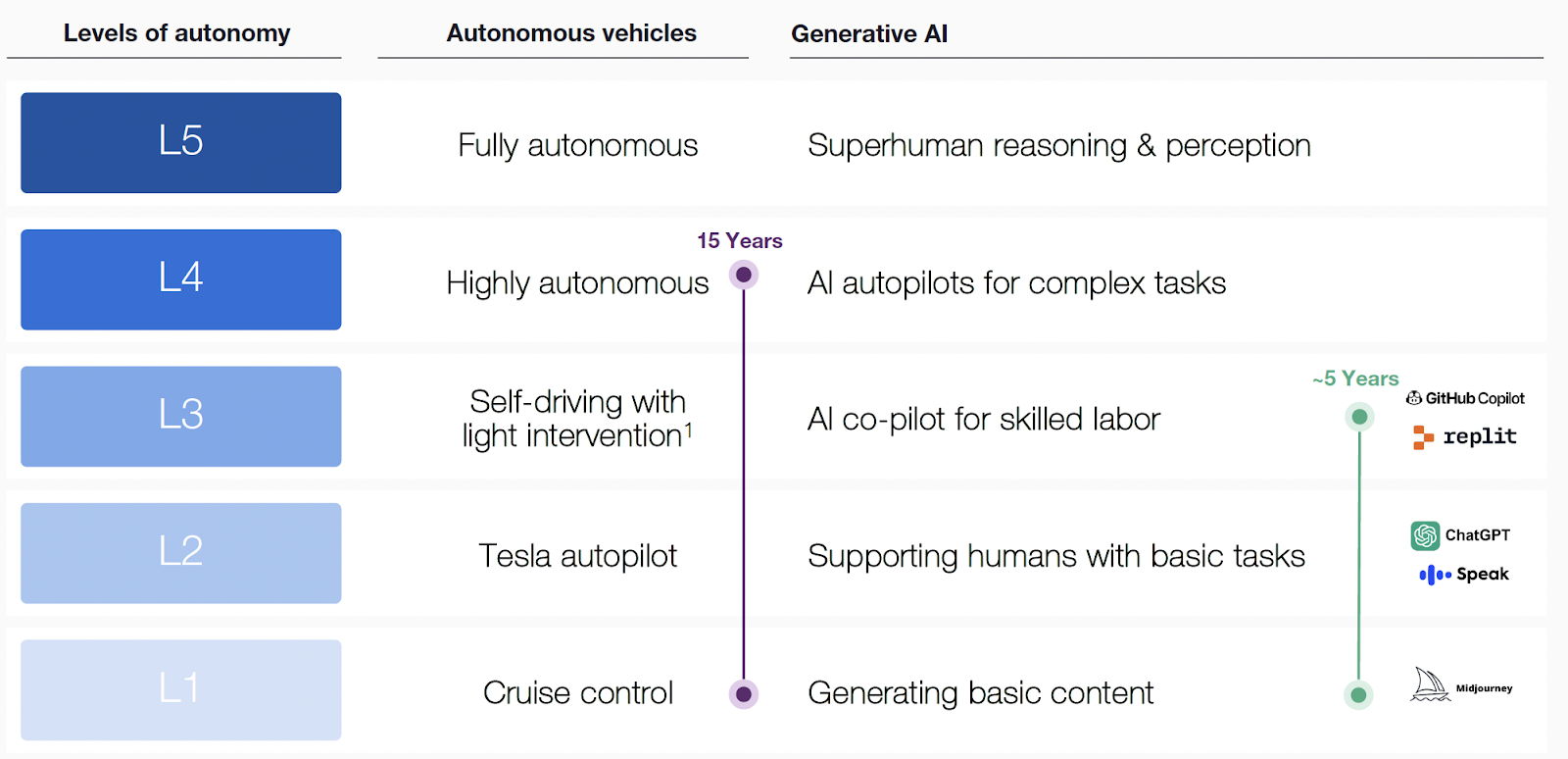
From the Coatue AI Report
From costs to compliance, profitability and board diversity, we outline key considerations for companies and industries taking Gen AI off the chatbot shelf and into real customer journeys.
1. Costs of competition. Competitive advantages will be defined by a new kind of PMF — product/purpose-model fit — whether it’s the open-source SeaLion (already being tested by companies like Tokopedia as reported in this Business Times article), OpenAI’s GPT (which we used for our website chatbot), or an entirely proprietary model (through data process automation enabled by companies like bluesheets). This means costs can rack up to keep up with competition on model superiority.
2. Costs of compute. Capital efficiency in driving adoption will be defined a new kind of CAC — Compute Acquisition Costs. With exponential productivity leaps, instead of scaling headcount to scale businesses, the use of AI would mean scaling compute instead. But integrating AI as a core component of the business requires managing high compute costs and high talent acquisition costs that come with maintaining use.
As Carro CEO Aaron Tan shares in a Straits Times piece on the future of AI-driven ecommerce in Singapore, “Unless the business is at scale, it is hard to cover the cost for AI.” Launching a chatbot is one thing, making sure it delivers consistently over time and evolves with customers and the business is another.
3. Costs of industry maturity. Capacity, compliance, and costs (3Cs) before applications (A). These 3C’s need to be made more accessible first before Gen AI’s application layer gains more widespread adoption. Parallels can be drawn with blockchain (as we discuss with Fazz Deputy CEO Tianwei Liu) and cloud when it comes to these 3Cs > A evolution.
Compliance in particular will be a question of which segments of customer journeys or features in products are best served by AI or not, as Ajaib CEO Anderson Sumarli illustrates in the case of stock investing.
4. Costs of profitability and UX. If there is PMF and CAC is managed, Gen AI can be at the intersection of driving unit profitability and improving customer experience for a company. The banking industry is ripe for this transformation.
Gen AI has the potential to decrease technical debt as well as improve the productivity of large customer-facing and white-collar workforces. McKinsey & Company reported that Gen AI could increase the overall industry revenue by US$340B and operating profits by 15%.
This opens up market opportunity for Gen AI for banking and financial services products. For example, WIZ.AI reports in this whitepaper that their generative AI solutions have helped increase customer reach by 70% and save 72% on costs for banking customer growth.
5. Costs of leadership. Board diversity will also be defined by AI expertise — and not just pure AI technical expertise, but also experts from specific sectors (like banking, supply chain, etc.) who have clearer view on the impact of AI on their industry or functional expertise.
As Yinglan shares in a feature by the Singapore Council for Board Diversity, “[Companies] also need a diversity of sector expertise simply because a lot of sectors are going to be disrupted by AI.”
Paulo Joquiño is a writer and content producer for tech companies, and co-author of the book Navigating ASEANnovation. He is currently Editor of Insignia Business Review, the official publication of Insignia Ventures Partners, and senior content strategist for the venture capital firm, where he started right after graduation. As a university student, he took up multiple work opportunities in content and marketing for startups in Asia. These included interning as an associate at G3 Partners, a Seoul-based marketing agency for tech startups, running tech community engagements at coworking space and business community, ASPACE Philippines, and interning at workspace marketplace FlySpaces. He graduated with a BS Management Engineering at Ateneo de Manila University in 2019.