OpenAI is just the tip of the iceberg
The rising global megatrend of AI-native enterprise applications distinguished themselves from the broader influx of AI-native apps such as Claude AI, Chorus.ai that emerged last year following the ascendance of OpenAI.
These pioneers stand out from the crowd, many of whom are still navigating the path to finding product-market fit.
Now with the help of generative AI providing competitive advantages to companies able to leverage the technology, AI-native enterprise applications are expected to tap into the markets long dominated by the legacy incumbents by transforming traditional services into software solutions, overhauling core workflows, and introducing innovative architectural paradigms that build upon the foundational AI model.
The blue ocean of AI native enterprise applications taking over SaaS
These companies formulated the blue ocean of AI-native enterprise apps, which has successfully unleashed unprecedented growth and surpassed historical benchmarks in the realm of Software as a Service (SaaS).
In the context of Southeast Asia, it is well poised for a significant increase by over 3.5x in AI investment from US$174 million in 2022 to US$646 million by 2026 according to an IDC report.
Moreover, a multitude of startups in the region are also in the race of developing AI-native products and services. Data from Tech in Asia indicates that 32 AI startups in Southeast Asia secured funding in 2022, marking the highest annual figure for the region to date. As of May 2024, in Southeast Asian, Singapore’s AI startups have already received the total funding of US$ 740 million.
This AI funding rush coincides with the rapid growth of the SaaS industry in Southeast Asia. Revenue in the Software as a Service market in SEA is projected to reach US$2.76bn in 2024, and grow to US$4.01bn by 2028, registering a 2024-2028 CAGR of 9.79%.
The strong investment in AI and significant adoption of Gen-AI in the region together with the robust growth of SaaS in Southeast Asia lay the groundwork for the emergence of AI-native enterprise applications from the region.
There are several global AI-native enterprise apps that stand out from the rest of the pack, paving the way to shape the product-market fit in the global scale and potentially in Southeast Asia level.
It is proven by Menlo Ventures’ study which indicates the growth trajectories of ten of these early frontrunners, whose rapid expansion surpassed all prior expectations.
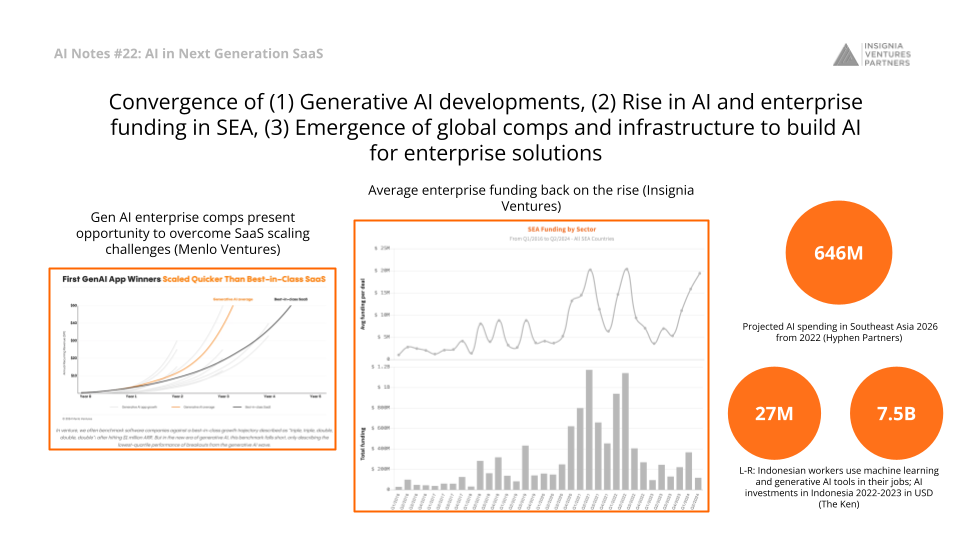
Convergence of (1) Generative AI developments, (2) Rise in AI and enterprise funding in SEA, (3) Emergence of global comps and infrastructure to build AI for enterprise solutions
5 key pillars for AI-native enterprise apps in Southeast Asia
While industry projections, comparables in the early global market, and birds eye view data paint a hopeful picture for Generative AI-enabled enterprise applications to take the region’s SaaS industry forward, it’s not quite a clear cut pathway.
From our analysis, we identify five key pillars that characterize this new wave of AI-native enterprise apps and how they would emerge from Southeast Asia.
(1) Develop solid use cases by focusing on the user problem and developing generative AI solutions to address top pain points:
First, substituting services with software.
Approximately 60% of Southeast Asia’s GDP is mainly contributed by the service sector. The prevalence of unstructured data and complex reasoning has hindered technological advancements in these areas.
However, Generative AI and data automation processing technology present opportunities to make sense of the unstructured data in these underserved sectors, revolutionizing Software as a Service (SaaS) with the concept of “Services-as-Software.” The former refers to software being integrated into a workflow or process to fulfill a specific service (1 to 1), whereas the latter refers to software that can provide services catered to a variety of specific needs or processes within a capability or function (1 to many).
Second, focusing on work with high value, volume, or labor shortages.
This second pain point is often the challenge pointed out with markets in Southeast Asia where the common perception is that labor markets dis-incentivize AI software adoption or even just any software adoption due to higher switching costs. But not all workflows and processes are limited by the cost arbitrage of labor.
AI can impact significantly in roles that are high-value (such as software engineers and lawyers), high-volume (such as customer service and business development representatives), or experiencing labor shortages (such as nurses).
(2) Develop a solution and tap into proprietary data.
First, target pattern-based workflows with significant engagement and usage.
A general framework on the different generative AI-driven solutions, organized on the human machine collaboration spectrum, is as follows:
- Co-pilots: that assist humans with ongoing tasks, in which a human must initiate prompts to a model when needed to complete specific tasks.
- Bots with human exception handling (e.g. supervisory AI agents): the AI will handle simpler tasks independently, and either ask for human intervention to approve how it handled such a task, or ask for human intervention on more complex tasks, or to handle exceptional cases.
- Autonomous AI agents (e.g. autonomous co-workers): the AI will carry out simple and complex series of tasks autonomously, without human intervention. Check out AI Notes on Autonomous AI Agents.
One effective strategy for AI applications is to target pattern-based workflows that exhibit regular usage and habitual engagement, rather than relying solely on data or network effects.
Co-pilots are currently the most commonly created and used solution, next to chatbots. According to Stanford’s “Getting Beyond the Sandbox: A Playbook for Developing Generative AI Solutions for Enterprise Applications”, there are co-pilots for software development, sales processes, marketing content, legal work product, medical diagnosis work, and more. Solutions that fall in category two and three are more experimental. However, there are developments with generative AI models and innovations by early-stage startups that are accelerating their commercial viability.
Autonomous generative AI agents is a recently emerging area. For example, Cognition’s product Devin is an autonomous software engineer released for customer beta testing and for demo purposes.
In its demo, Devin is provided abstract goals and would independently reason on paths to achieving such goals, including how to complete projects with software-related tasks and challenges. More product experiments and research are needed in this space to determine how effectively an agent can take actions to fully complete projects, but these are early signs pointing towards the prevalence of this type of engagement and collaboration between humans and machines.
Second, tap into proprietary data.
80% of enterprise data remains inaccessible due to being trapped in unstructured file formats or document repositories like email inboxes. By leveraging Large Language Models (LLMs), AI-native applications can unlock this data, establishing proprietary data advantages over time.
There are various methods to use proprietary data to customize generative AI solution outputs: context window, retrieval augmented generation (RAG), fine-tuning a pre-existing model, or pre-training a model from scratch with one’s own data.
Learn more about how bluesheets helps companies make sense of unstructured data and their data workflows:
(3) Leverage zero marginal cost creation
Generative AI enables unlimited scaling of written content, images, and potentially even software and products, facilitating creation possibilities across various enterprise functions, from cold sales outreach to personalized marketing and compelling visual assets.
(4) Develop and innovate where the dominant players aren’t, can’t, or won’t.
Identify areas where Generative AI can introduce new capabilities, incumbents are slow to adapt, or the dominant player lacks foresight regarding AI possibilities.
(5) Excel with compound AI rather than models
The most successful AI applications add value at the data and infrastructure levels, leveraging innovative architectural approaches such as chain-of-thought, tool usage, agents, and appropriate model selection for specific functions, rather than relying solely on a single monolithic model.
Learn more how companies like WIZ.AI have done this to great effect: